"Fair Personalized Learner Modeling Without Sensitive Attributes"—a research paper by Xu Hefei (supervised by Prof. Wu Le) and collaborators from our school’s Media Computing Lab—proposes FairWISA, an innovative method for achieving fairness in personalized learning without relying on sensitive attributes. No sensitive data? No problem. FairWISA enables equitable modeling anyway. We look forward to its future applications in educational systems. The paper has been accepted by The ACM Web Conference (WWW), a CCF-A ranked top-tier conference.
Paper Title: Fair Personalized Learner Modeling Without Sensitive Attributes
Authors: Hefei Xu, Min Hou, Le Wu, Fei Liu, Yonghui Yang, Haoyue Bai, Richang Hong, Meng Wang
Paper Link: https://openreview.net/forum?id=6LdQbh1dg0#discussion
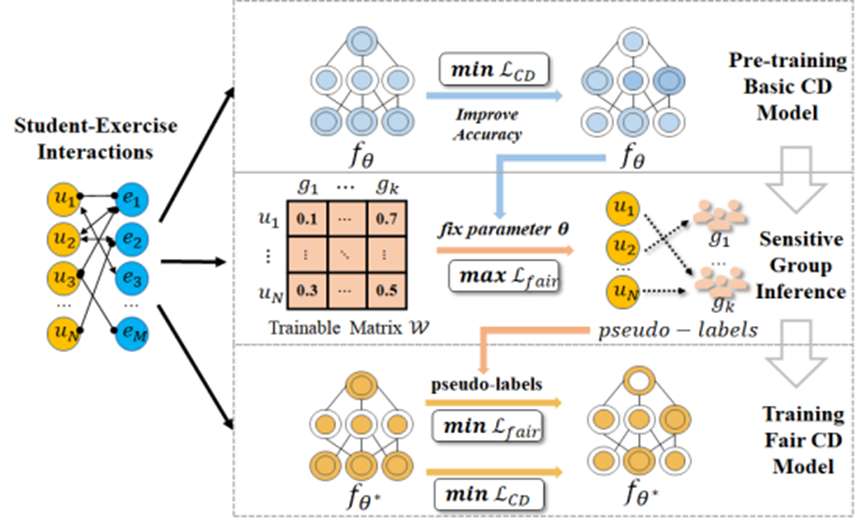
Figure 1: The framework of FairWISA
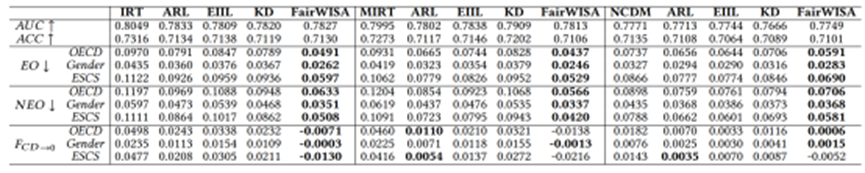
Table 1: Comparison of different approaches on PISA. OECD, Gender, and ESCS serve as sensitive attributes, dividing data samples into two groups for fairness evaluation. Bolding signifies the best performance among all methods

Table 2: Comparison of different approaches on PISA challenging test. OECD, Gender, and ESCS serve as sensitive attributes, dividing data samples into two groups for fairness evaluation. Bolding signifies the best performance among all methods

Figure 2: Comparision of Reg_EO and FairWISA. FairWISA-IRT (FairWISA-MIRT) and Reg_EO-IRT (Reg_EO-MIRT) denote FairWISA and Reg_EO methods implemented with IRT and MIRT as backbones, respectively. Plot (a) shows the performance in terms of accuracy, while Plots (b), (c), and (d) show the performance in terms of fairness
Abstract: Personalized learner modeling uses learners’ historical behavior data to diagnose their cognitive abilities, a process known as Cognitive Diagnosis (CD) in the literature. This is a fundamental yet crucial task in web-based learning services, such as learning resource recommendation and adaptive testing. Previously, researchers discovered that models improperly correlate learners’ abilities with their sensitive attributes, resulting in unfair diagnoses for learners from different sensitive groups (e.g., gender, region). Given the input of sensitive attributes, researchers proposed decorrelating these attributes from the modeling process, demonstrating improved fairness results. However, privacy concerns make collecting sensitive attributes impractical. This challenge is compounded by the presence of multiple sensitive attributes, making fairness improvement under any of them difficult. In this paper, we explore how to achieve fair personalized learner modeling without relying on any sensitive attribute input. Specifically, we first introduce a novel fairness objective tailored for personalized learner modeling. We then propose a max-min strategy that facilitates both potential sensitive information inference and fair CD modeling. In the max step, we propose a pseudo-label inference method based on maximizing the designed fairness objective. Given these pseudo-labels, the min step involves retraining a fair CD model by minimizing the designed objective. Additionally, we provide a theoretical guarantee that implementing our proposed framework reduces the upper bound of fairness generalization error. Extensive experiments demonstrate that the proposed framework significantly outperforms existing methods in terms of fairness and accuracy. Our code is available at https://anonymous.4open.science/r/FairWISA-40C6/.
The ACM Web Conference (abbreviated as WWW) is one of the world's premier annual academic conferences, focusing on the development of the World Wide Web and related technologies. Organized by the International World Wide Web Conference Committee (IW3C2) in collaboration with ACM SIGWEB, the conference is held annually, rotating among North America, Europe, and Asia. Recognized as a Class A international academic conference by the China Computer Federation (CCF), the WWW conference enjoys an outstanding reputation in academia. With an acceptance rate of only 19.8%, it reflects the rigorous review standards and high-quality academic research showcased at the event.